Smartphone-based Flow Sensing for Prosthetic Heart Valve Monitoring
Bernhard Vennemann, Dominik Obrist, and Thomas Rösgen
Abstract
Cardiovascular diseases are the leading cause of death in Switzerland [1]. Many of these diseases are associated with a malfunctioning aortic valve (AV) [2]. The failing native AV is routinely treated with replacement with a bioprosthetic heart valve (BHV). However, their durability is limited and often less than the patient’s life expectancy. Close monitoring of BHV function can therefore help to improve long-term outcomes by early detection of adverse events.
A wireless and batteryless implantable blood flow monitor
We developed a blood flow sensing implant in the shape of a perivascular cuff which attaches to the ascending aorta [3,4]. Recording and automated analysis of the characteristic patterns of blood flow downstream of the valve can yield important insight into the state of the AV.
The implant is based on the working principle of a magnetic flow meter that was modified to allow for ultra-low power operation. Near field communication technology is used to enable wireless, transcutaneous communication with the patient’s smartphone and to power the implant through inductive energy transfer.

Automated diagnostics using machine learning
A machine learning framework was developed that is used to automatically analyze the sensor recordings in an unsupervised fashion and to detect adverse cardiovascular events that are indicative of a malfunctioning valve [5].
After BHV implantation and after a run-in period, flow recordings are obtained from the sensor which are then used to train a patient-specific statistical baseline model of the characteristic flow patterns of a functioning BHV. Flow recordings during the successive monitoring phase are compared to this baseline to automatically detect flow behavior which is indicative of an underlying valvular pathology. Heart valve performance is quantified using a diagnostic index that reflects the current state of the BHV and that can be monitored over time to reveal progressive AV degradation.

Validation
An in-vitro test setup, capable of replicating the flow- and pressure conditions in the human body was used to test the ability of the monitoring system to automatically detect valvular malfunction. Various degrees of aortic regurgitation (AR) were deliberately introduced using tube-shaped inserts in the center of the BHV. Flow recordings without insert were used to train the machine learning model that was subsequently utilized to automatically detect a leak in the valve.
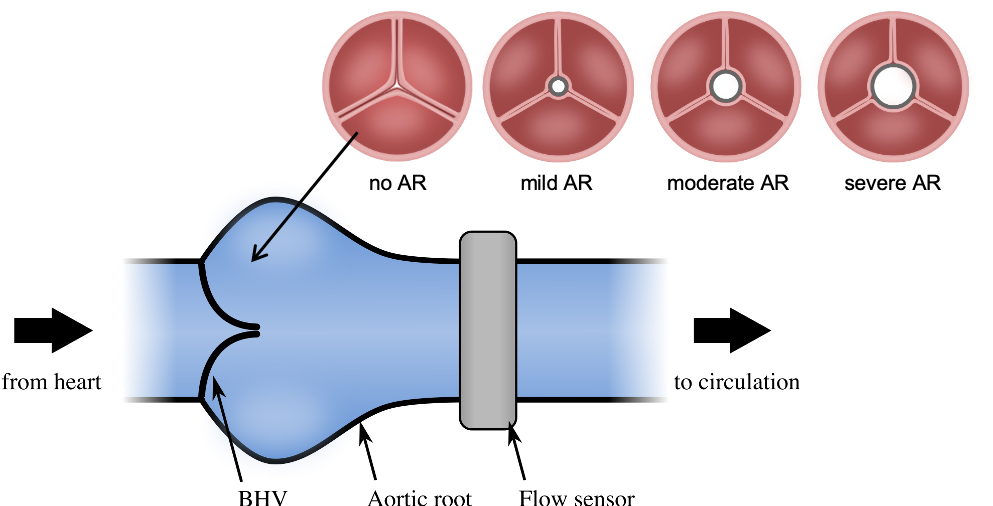
The monitoring system was successful in detecting the valvular defects with a sharp decrease in the diagnostic index for the regurgitant valves which confirms that AV degradation may be automatically detected using flow sensing implants and unsupervised machine learning models.
Sensor implants that are coupled with the power of machine intelligence provide the clinician with novel tools that have the potential to improve healthcare, while reducing workload through automation of diagnostic tasks.
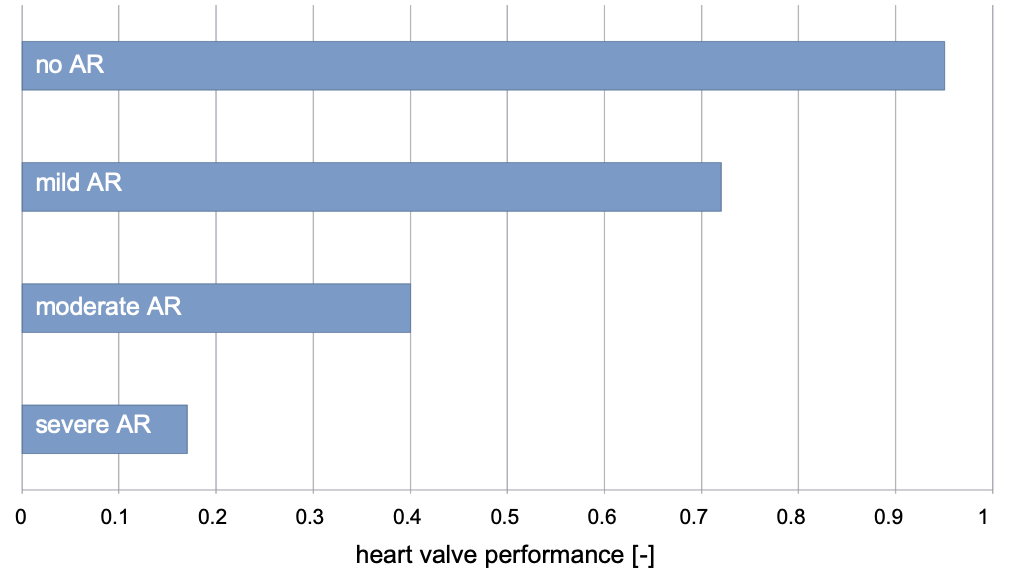
References
[1] Junker, C., Federal Statistical Office, 2015.
[2] Otto, C., Bonow, R., Elsevier, 2014.
[3] Vennemann, B., 2019. doi:10.3929/ethz-b-000334207
[4] Vennemann B., Obrist D., Rösgen T., 2020 PLoS One
[5] Vennemann B., Obrist D., Rösgen T., 2019 PLoS One